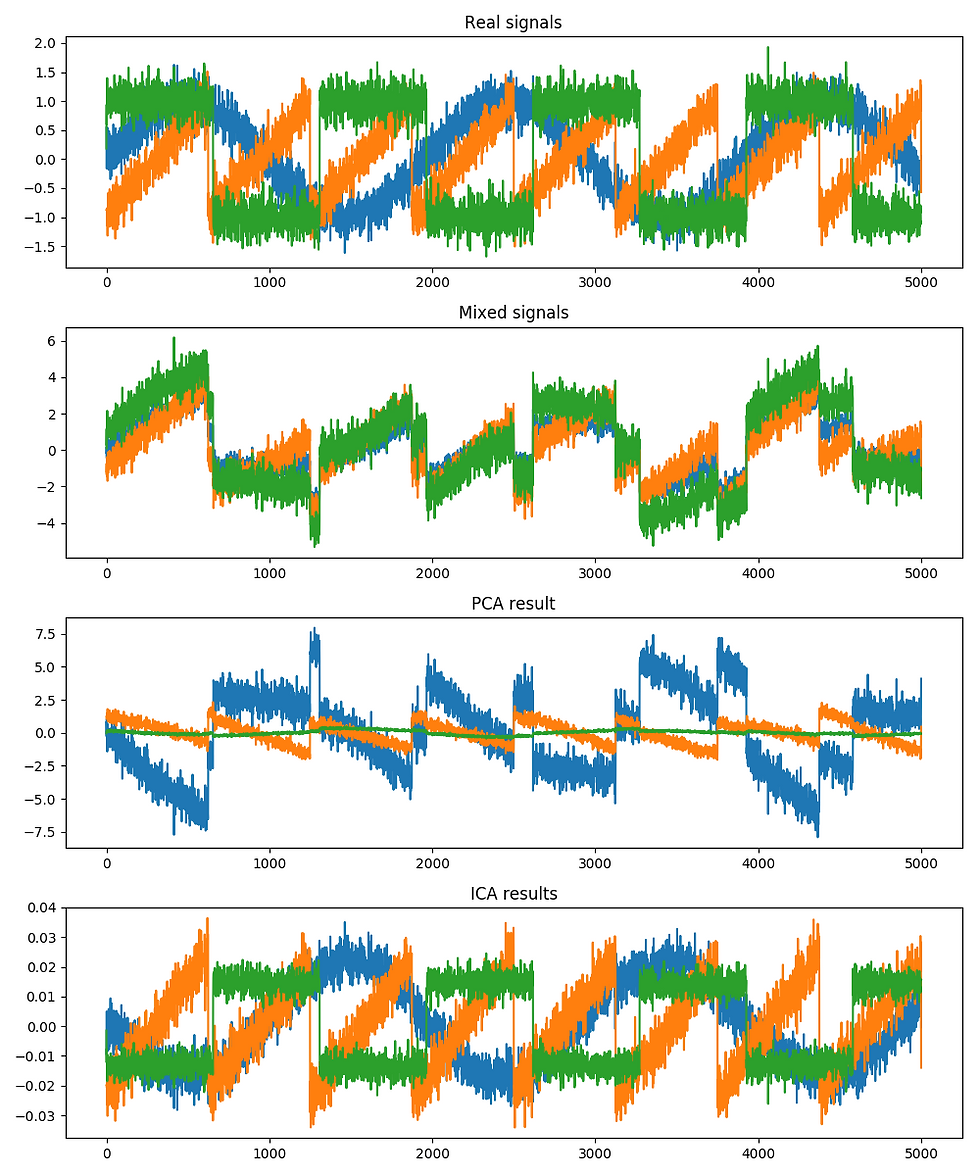
Preference Learning Toolbox (PLT) Crack X64 The PLT toolbox allows you to apply preference learning algorithms to data from a wide variety of sources, e.g., ratings, a ranking, probability-distribution, or decision tables. Concepts: - Preference Learning - Classification Learning - Preference Learning Methods - Multi-Armed Bandits - Markov Games - Sequential Decision Processes Preference Learning Algorithms: PLT provides a wide range of preference learning algorithms and allows to model complex real-world applications, e.g., games, scheduling, recommendation, and optimization. Preference Learning Examples: - Contingency and Pareto Efficiency: Comparative Ranking and Multi-Objective Optimization - Preference Learning with Learning to Rank: Ranking Rating Sets and Recommender Systems - Preference Learning in Economics: Political Preferences - Preference Learning with Attribute Incomplete Data: A Multi-Armed Bandit Example - Rival Preferences and Reasoning Models - Sequential Decision Processes Applications: - Numerical Problem Solving: Preference-Learning Algorithms - Games: Preference Learning, Convergence Analysis, and Computational Complexity - Scheduling: Preference Learning in Non-preemptive Scheduling - Intelligent Agents: Multi-Objective Optimization in Games with Incomplete Information - Recommendation: Preference Learning in the Recommender System Preference Learning Methods: Preference Learning Methods in PLT is an enhanced version of the related Preference Learning Methods package in MATLAB, which is extended to the addition of multi-objective preference learning, learning to rank, sequential decision processes, and multi-armed bandits. PLT provides pre-made matrices for the analysis of some of these methods, including Random Forests, Naïve Bayes, and Conditional Decision Trees. PLT was tested on real world data and gives users the flexibility to apply preference learning methods using their own data. Multi-Armed Bandit: The PLT multi-armed bandit method provides a generalized utility model that accommodates many types of decision problems, and is well suited to problems where rewards come in discrete (possibly asymmetric) form. Many real-world problems involve decisions where a series of actions with varying possible outcomes are performed sequentially. For example, we might choose to buy a book, and we might choose the genre of a book that we like best. If we chose to buy a Preference Learning Toolbox (PLT) License Code & Keygen For Windows The Toolbox is a Java application and contains 10 tutorial examples. In the first example, we learn about preference learning in a simple scenario where we have information about the preference of customers for products. In the following examples, we learn about preference learning using different objects and types of data (sets of numbers, categorical data and texts). Usage: Clicking on a tutorial example takes you directly to that tutorial example. In the example, you can use the "help" button to help you learn the basic concepts behind the tutorial. Click on "readme.txt" to find the tutorial text and at the bottom of the page you will find the examples of the tutorials. User feedback: We are looking forward to your feedback. Please do not hesitate to contact us for additional information: info@preference-learning.com License: This toolbox is distributed under the GNU General Public License. Developers: Preference Learning Toolbox developers are Johan Westerhoff and Sander Kuyken. Acknowledgments: You may wish to acknowledge the following individuals for their assistance in developing the toolbox. - Jon Phelan - Graeme Lindsay - Mark Stamper - Jarle Friis - Sjors van Zweden - Frank Wolber - Chris Pedrick - David Egan - Gabriel Swierstra - Steve Grimes - Sjoerd Winter - Andy Johnson - Jan-Willem van den Bosch - Raphael Horn - Mijnoula Lefkimmiatis - Rachid Ait Ouannane - Guillermo Gómez-Barroso - Sylvain Huet - Jason Wang - Pierre Collin - Till Gruber - Michael Schranz - Carl Lianes - Tom DeWeese - Tom Rominger - Jochen Eisel - Andreas C. Krause - Marc Schott - Adnan Chemirani - Martin Braun - Bernhard Heinrich - David Egan - Philippe Thibaud - Olivier Favre - Mija Kiribati - Jean-Marc Ganascia - Edgar Arenas - Rafael Martins - Gerald Poynder - Rudolf Wittink - Robert Piché - André Collin - Oliver Seitz - Daniel Neis - Daniel Cremers - Christoph Monien - Toby Walsh - Adrien Friseur - Werner Kün 1a423ce670 Preference Learning Toolbox (PLT) With Full Keygen 2022 * Apply preference learning to generate a decision-making machine that can make choices autonomously * Compare the performance of different algorithms * See how preference learning works Version Information: * Preference Learning Toolbox is provided by KeyMACRO * A full keycode-gen program is provided * Generate / Recognize Mac-Keycodes * Generate / Recognize PC-Keycodes * (Reference to Mac-keycodes to PC-keycodes and vice versa) An easy-to-use JAVA-based utility that can recognize any keyboard layout, including legacy and ANSI standard Macintosh keyboards. Works on Windows too, but needs WinModem and Mac Modem drivers. No Mac Modem is needed on Mac for all input modes (both ANSI and Legacy). KeycodeGen2 is capable of inputting a lot of keyboard layouts, like ANSI/ASCII, AltGr, German, English, Japanese, and many others. Currently the following keyboard layouts are supported: Asc ANSI Mac keyboard layout ANSI Mac keyboard layout (with Shift and Control keys) ANSI Mac keyboard layout (without Shift and Control keys) ANSI Windows keyboard layout ANSI Windows keyboard layout (with Shift and Control keys) ANSI Windows keyboard layout (without Shift and Control keys) ANSI Windows keyboard layout (no Shift) ANSI Windows keyboard layout (no Shift, no Control) ANSI Windows keyboard layout (no Shift, no Control, no AltGr) ANSI Windows keyboard layout (no Shift, no Control, no AltGr, no Control, no AltGr, no Shift, no Control) ANSI Windows keyboard layout (no Shift, no Control, no AltGr, no Control, no AltGr, no Shift, no Control, no AltGr) ANSI Windows keyboard layout (no Shift, no Control, no AltGr, no Control, no AltGr, no Shift, no Control, no AltGr) ANSI Windows keyboard layout (no Shift, no Control, no AltGr, no Control, no AltGr, no Shift, no Control, no AltGr) ANSI Windows keyboard layout (no Shift, no Control, no AltGr, no Control, no AltGr, no Shift, no Control, no AltGr) ANSI Windows keyboard layout (no Shift, no Control, no AltGr, no Control, no AltGr, no Shift, no Control What's New In Preference Learning Toolbox (PLT)? System Requirements: Minimum: OS: Windows 7 64-bit or later Processor: Intel Core i5-6200, AMD Phenom II x6 1100T, or better Memory: 8GB RAM Hard Drive: 25GB of available space Recommended: Processor: Intel Core i7-6700K, AMD Ryzen 7 1700X, or better Memory: 16GB RAM Graphics: Nvidia GeForce GTX 1080,
Related links:
Comments